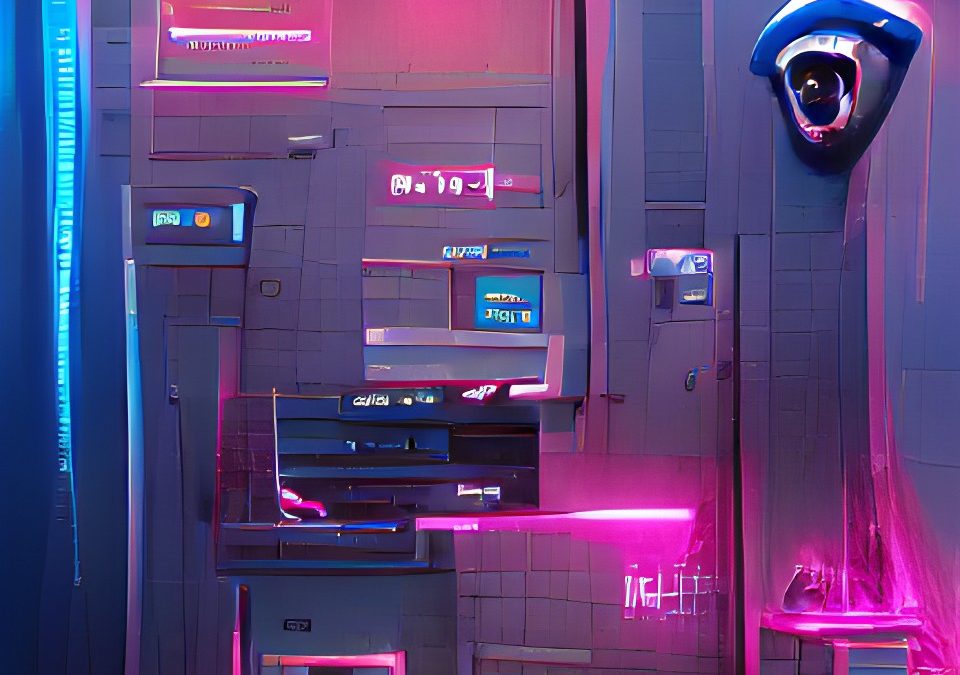
Guard your privacy with Spiki’s speech command recognition
Where does your voice assistant keep your data?
Voice assistants make our lives so much easier: simply activating our lights, dropping a phone call or playing our favourite music by just using speech commands, hands free. Several devices, assistants and routers provide us with formidable convenience at our spoken request. Of course, an active Internet connection is required for that. Sometimes different web apps come into play to further finetune and navigate tasks. It’s all quite simple and intuitive. Nothing to worry about.
Really?
Do you value, beside the mere convenience of using voice assistants, your data privacy and security? Where does your voice assistant keep your data? Does it listen to you ALL THE TIME, storing, processing or even sharing the most personal details?
Risks of online voice processing devices
Indeed, every user shares a great deal of information, even when only talking privately at home. Usually, these recorded data are stored and processed in a cloud. This poses a risk to security, safety and privacy.
- Firstly, hackers and cybercriminals can gain access to data stored in a cloud system. Sensitive information could get into the wrong hands, e.g. access codes, passwords, financial or health information etc.
- Secondly, a user’s voice is regarded as biometrical data which can identify a human being. Voice recordings stored locally are not in danger, but stored in a cloud they could get subject to abuse. Big tech corporations frequently store data generated from voice recognition devices in a cloud, analyse and use them in order to improve their software or their advertising.
- Here is a prime example: “Amazon.com Inc. must produce millions of documents in response to discovery requests in a potential class action over the marketing of its Alexa-enabled devices and their recording of users’ conversations, a federal judge ruled”, reports Christopher Brown on Bloomberg Law (see article).
- Improper or not – some big tech companies already offer their users to opt in and allow their voice recordings to be stored, while others require them to actively opt out if they do not want this. Feel free to check for yourself the terms of use of your trusted voice assistant to stay in control.
- Any company’s information is a top security priority. In the wake of the COVID pandemic, many corporate meetings had to be transferred from personal to the online world. Video conference tools mainly use end-to-end encryption, which secures privacy and security of the online communication. Still, the encrypted video and audio content is stored in the cloud…you see where this is going, check first bullet point.
In summary, data privacy and cyber security are major challenges for both private and corporate users of voice processing software and devices using speech commands.
How to guard your privacy and security
There is only one reliable solution in order to protect your sensitive information: to store and process voice data locally or at the edge, which means closer to the device used. Beside keeping data secure, this would also solve latency issues slowing down the processing of data stored in a remote cloud server.
Spiki’s voice command recognition keeps your data locally and guards your privacy.
Relying on Spiki’s robust voice command recognition, you eliminate privacy and compliance risks and keep stored data safe. Reducing latency will reduce computation time. Since our IP can run on any third-party hardware, you save time and implementation costs. Spiki’s voice recognition is offline, to keep your private details private.
- It works without Internet connectivity
- It ensures privacy of customer data
- It is usable in noisy environments, robust and reliable to fulfil your specific requirements
- It requires no additional hardware (e.g., Alexa).
Excited? Get in touch for receiving a demonstration!